Leveraging Machine Learning for Exchange Rate Prediction: A Business and Financial Management Perspective in Nigeria
Keywords:
Machine learning, Logistic linear regression, Support vector machine,, Random forest, XGBoost algorithms, Exchange rateAbstract
Purpose
The continuous availability of historical data for asset prices propelled more attention of researchers to use analytical algorithms to study the evolution of prices. This paper aims to use four machine learning algorithms to forecast the exchange rates in Nigeria.
Methodology
The paper employs Logistic Linear Regression, Support Vector Machine, Random Forest, and XGBoost algorithms to predict the univariate time series of Nigeria's exchange rate against the US dollar, using both hourly and daily data.
Findings
The findings indicate that the Random Forest (RF) model outperforms other approaches in predicting Nigeria’s exchange rate against the US dollar, demonstrating the lowest prediction errors (MAE, MSE, RMSE, and MAPE). RF remains the most accurate model across both hourly and daily frequencies, with XGBoost emerging as the second-best performer.
Conclusions
This study applies machine learning models to enhance exchange rate prediction, demonstrating that the exchange rate series is not sensitive to data periodicity. The findings provide valuable insights for stakeholders in the foreign exchange market, aiding policymakers in selecting the most accurate forecasting techniques.
Downloads
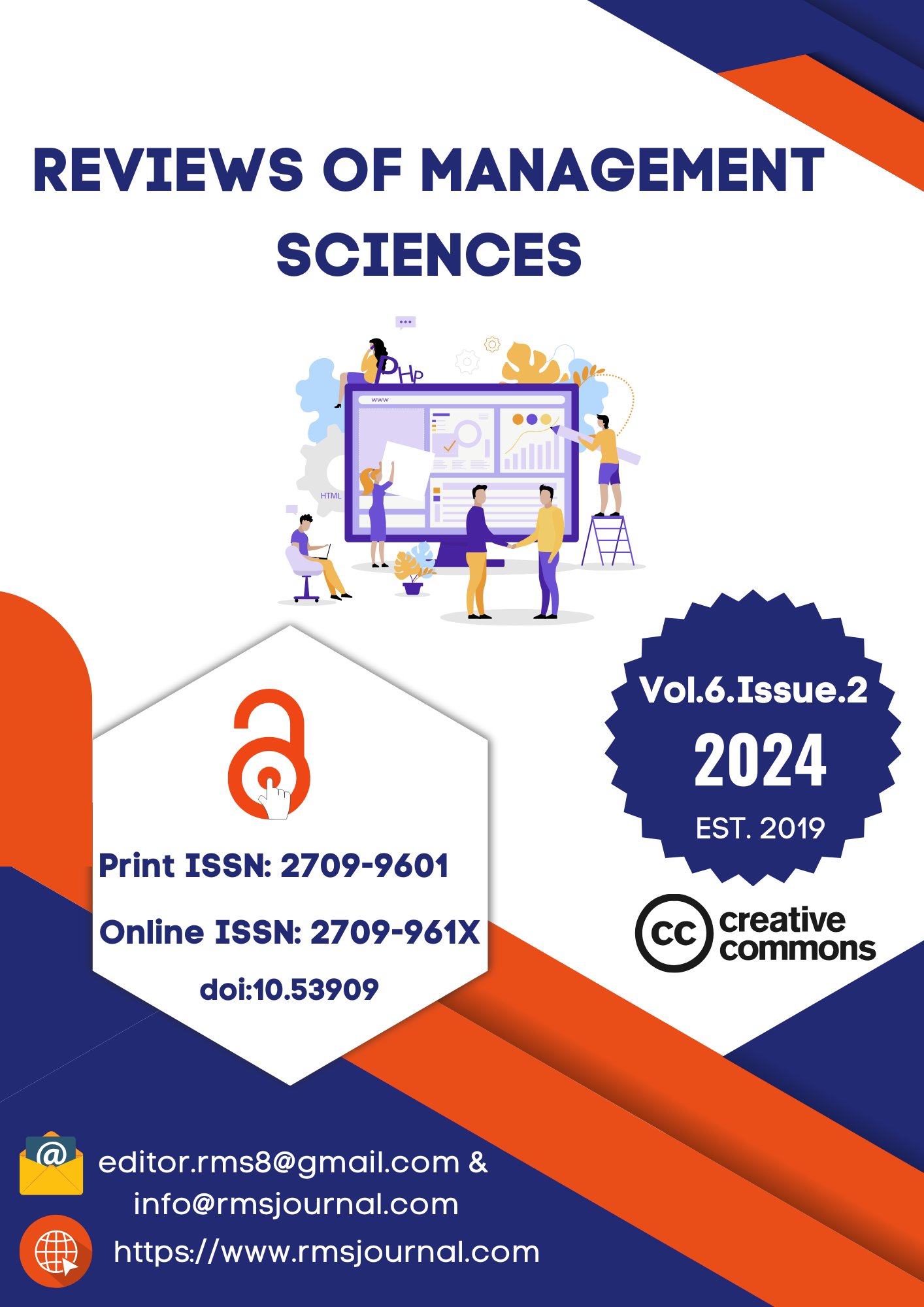
Downloads
Published
How to Cite
Issue
Section
License
Copyright (c) 2025 Authors retain copyright to the content of the articles. Open access articles can be published under the Creative Commons Attribution (CC BY) 4.0

This work is licensed under a Creative Commons Attribution 4.0 International License.
The open-access articles in this journal are licensed under the terms of the Creative Commons licenses (CC BY 4.0).